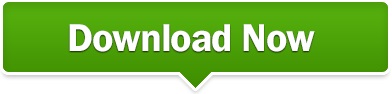
Thus, the coefficients of the other factors remain the same, and model analysis becomes much easier. Instead of building completely different models, multilevel regression changes the coefficients of only some parameters in the model for each level of random effects. Multilevel models can remove this trouble. In that case, how can we generalize the results and say if Technique is really a significant factor? Some may have significant effects of Technique, and some may not. With the example we used above, we would have 10 models in total. If we build a separate model for each participant, for example, analysis would be very time-consuming. For the factors in which we want to take individual differences into account, we treat them as random effects and build each model for each level of these factors. The previous section gave you a rough idea of what multilevel models are like. But I think this exaggerated explanation well describes how multilevel regression is different from simple regression, and is easy to understand. What multilevel regression actually does is something like between completely ignoring the within-subject factors (sticking with one model) and building a separate model for every single group (making n separate models for n participants). In this way, we can also consider individual differences of the participants (they will be described as differences of the models). Thus, in this example, instead of having one linear model, you will build 10 linear models, each of which is for each participant, and do analysis on whether the techniques caused differences or not. Multilevel regression, intuitively, allows us to have a model for each group represented in the within-subject factors. The linear regression above tries to represent the data with one line, and unfortunately it aggressively aggregates such differences which may matter to your results in this case. Some participants had similar performance with both techniques, and some had much better performance with one technique. Or the differences of the techniques might have caused different levels of the effects depending on the participants. For example, some participants are more comfortable with using computers than the others, and thus, their overall performance might have been better. However, this analysis does not fully consider the experiment design you had: the differences between the participants. If you do not consider the “Participant” factor, you will do a linear regression analysis where your independent variable is Technique, and if its coefficient is non-zero, you will argue that the difference of the techniques caused some differences in the performance time.
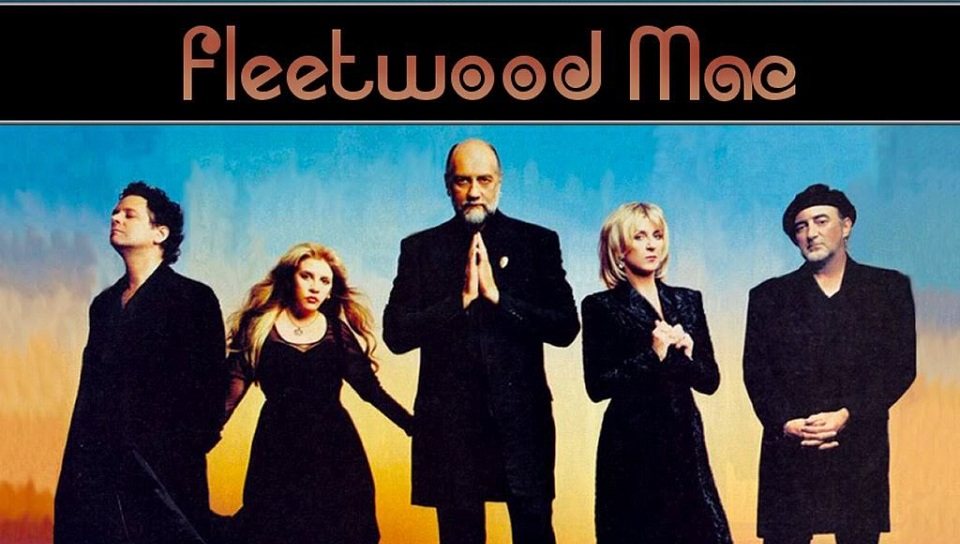
In your experiment, 10 participants performed some tasks with both techniques thus, the experiment is a within-subject design. Let's think about a very simple experiment: Comparing two techniques: Technique A and Technique B. So, I won't go into detailed discussions about how we should consider these factors.īefore jumping into examples of multilevel linear models, let's have a high-level understanding of multilevel linear models. I explain what “random effects” and “fixed effects” (opposite to random effects) mean in this page however, people say different opinions about them (as Gelman and Hill's book explains). In this wiki, I follow Data Analysis Using Regression and Multilevel/Hierarchical Models by Andrew Gelman and Jennifer Hill.
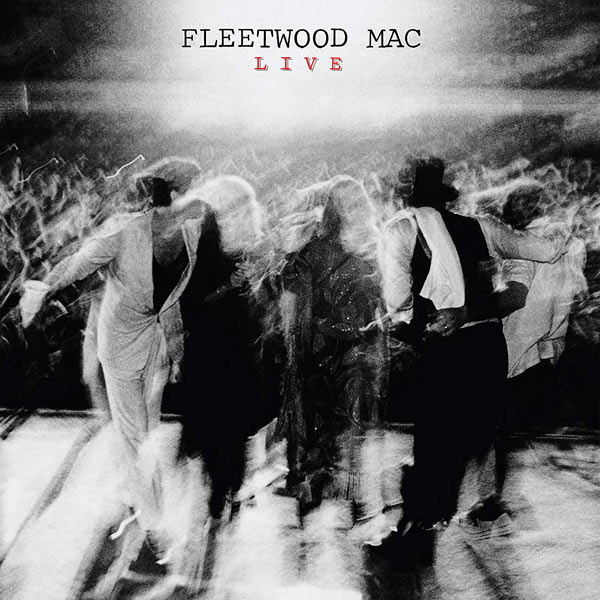
The term of “random effects” is often confusing because it is used to mean different things. Very roughly speaking, it is a repeated-measure version of linear models or GLMs.Ī multilevel model is often referred as a “hierarchical,” “random-effect” or “mixed-effect” model. Multilevel models can accommodate such differences. These models are also used for prediction: Predicting the possible outcome if you have new values on your independent variables (and this is why independent variables are also called predictors).Īlthough these models are powerful for analyzing the data gained from HCI experiments, one concern we have is that they do not carefully handle “repeated-measure”-ness ( e.g., individual differences of the participants). Linear Models and Generalized Linear Models (GLM) are a very useful tool to understand the effects of the factor you want to examine.
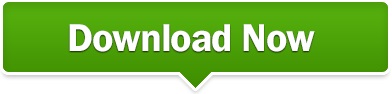